Impulse Project 12: AURORA - Functionalsafety for Automotive Objekt Recognition of Optical sensors by state monitoring of CameRAs
Vehicle sensors, crucial for safety-relevant environment detection, degrade over time. The AURORA project, led by Prof. Elger, is cantered on Predictive Health Monitoring to anticipate this degradation, ensuring enhanced safety. Car2X communication, a pivotal feature, provides comprehensive data, both internal and external. The project's ambition is not only to refine the SelfX diagnostic function but also to use advanced machine learning algorithms. These algorithms are designed to identify potential sensor malfunctions early, further safeguarding vehicular operations.
WP1 and WP2: Development of Monitoring Systems for Lens and Optical Modules
A comprehensive measurement system is in development, encompassing both lens condition and optical module monitoring. These systems are designed to track key indicators, covering aspects like sharpness and aberrations, especially under temperature changes in aged components. Based on the data generated in these work packages WP4 will train an algorithm to predict remaining useful life (RUL) of lenses and optic modules.
WP3 Influence of Camera Properties on Object Detection
This package investigates the influence of camera condition on image defocusing and object detection. Optical simulations are conducted in a DOE study and the corresponding effect or state of the art object detection algorithm is tested on these simulated images. A validation study is performed moving the lens on the optical axis to emulate defocus in the system and capturing scenarios involving vulnerable road users.
WP4 Condition Monitoring of Optical Modules
An algorithm will be developed which assesses the optic module's condition based on data from WP1 and WP2. The key indicators of lenses and optical modules determine their health and RUL.
WP5 Assembly of the Lens and Lens Holder PCB Package
A mounting system connects a lens and its associated Imager-PCB package using passive optical alignment. The lens aligns with a hexapod and attaches to the package. This helps develop simplified assembly process for optic modules.
WP6 Field Sharpness Monitoring
Optical modules from work package 3.2.5 will be integrated into a research vehicle. These modules will be continuously read out, with data transferred to a cloud. Using this data and algorithms, key indicators will be determined. Machine learning algorithms from WP4 trained for safety-relevant metrics would evaluate the optic module health.
Based on lab data a “0 lifetime model” is installed on vehicles which assesses state of sensors based on health indicators. This data is sent to “reliability cloud” where a trained algorithm detects anomaly and estimates lifetime “1 model”. Relevant data is extracted and used in the training data pool replacing the “0 model” with the “1 model”. Failure and lifetime data is shared with OEM and manufacturer for improvement and also shared with the vehicle user. Lifetime model is periodically trained and updated as “n-model”.
Contact
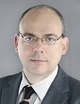
Prof. Dr. Gordon Elger
Phone: +49 841 9348-2840
Room: A114
E-Mail: Gordon.Elger@thi.de
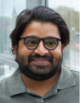
Amit Pandey, M. Sc.
Phone: +49 841 9348-5175
E-Mail: Amit.Pandey@thi.de